
InMemoriam
Reddit Repost Bot
★★★★★
- Joined
- Feb 19, 2022
- Posts
- 9,432
Toward Stopping Incel Rebellion: Detecting Incels in Social Media Using Sentiment Analysis
May 2021DOI:10.1109/ICWR51868.2021.9443027
Conference: 2021 7th International Conference on Web Research (ICWR)
Authors:
Abstract
Incels, which stand for involuntary celibates, refer to online community members who identify themselves as individuals that women are not attracted to them. They are usually involved in misogyny and hateful conversations on social networks, leading to several terrorist attacks in recent years, also known as incel rebellion. In order to stop terrorist acts like this, the first step is to detect incels members in social networks. To this end, user-generated data can give us insights. In previous attempts to identifying incels in social media, users’ likes and fuzzy likes data were considered. However, another piece of information that can be helpful to identify such social network members is users’ comments. In this study, for the first time, we have considered users’ comments to identify incels in the social networks. Accordingly, an algorithm using sentiment analysis was proposed. Study results show that by implementing the proposed method on social media users’ comments, incel members can be identified in social networks with an accuracy of 78.8%, which outperforms the previous work in this field by 10.05%accuracy of 78.8% impressive
2021 7th International Conference on Web Research (ICWR)
169
978-1-6654-0426-6/21/$31.00 ©2021 IEEE
Toward Stopping Incel Rebellion: Detecting Incels
in Social Media Using Sentiment Analysis
Mohammad Hajarian*
Department of Computer Science, Universidad Carlos III de
Madrid, Leganes, Spain
[email protected]
Zahra Khanbabaloo
Department of Computer Engineering, Basir Institute of
Higher Education, Abyek, Iran
[email protected]
Abstract—Incels, which stand for involuntary celibates,
refer to online community members who identify themselves as
individuals that women are not attracted to them. They are
usually involved in misogyny and hateful conversations on
social networks, leading to several terrorist attacks in recent
years, also known as incel rebellion. In order to stop terrorist
acts like this, the first step is to detect incels members in social
networks. To this end, user-generated data can give us insights.
In previous attempts to identifying incels in social media,
users’ likes and fuzzy likes data were considered. However,
another piece of information that can be helpful to identify
such social network members is users’ comments. In this study,
for the first time, we have considered users’ comments to
identify incels in the social networks. Accordingly, an
algorithm using sentiment analysis was proposed. Study results
show that by implementing the proposed method on social
media users’ comments, incel members can be identified in
social networks with an accuracy of 78.8%, which outperforms
the previous work in this field by 10.05%.
Keywords— Social Networks; Social Media Comments;
Incels; Sentiment Analysis; Detection Method.
I. INTRODUCTION
April 23, 2018, shortly after Alek Minassian posted on
his Facebook, “incel rebellion has started,” the Toronto van
attack began, and ten innocent people died. Tracing back the
roots of this tragedy, we can see that with increasing social
media usage, the misogyny talks generated by different users
have been raised [1], and these types of comments inspired
Alek Minassian and several other people. As a result, if
social media does not control these comments, this can lead
to devastating societal problems [2]. Incels stand for
involuntary celibates [3], and incel communities usually
include single male users who think women are not attracted
to them. In some of the incel talks, people might become
radical and involve in misogyny, hateful, sexism, or racism
talks.
Social media play different roles in human life, and
people may use them for various reasons, from staying
connected with friends and family to early warning
applications [4, 5, 6, 7, 8]. However, some people may use
social networks to fill their loneliness [9, 10, 11, 12],
including incels. In this context, social media users’
comments can show people’s feelings and emotions [13, 14,
15, 16]. Users’ comments have been the subject of many
studies for different purposes. From showing relevant
advertisements based on the uses’ emotions [17, 18] to detect
fake news [19, 20, 21], the users’ comments are valuable
sources of information that reveal many facts about the users
[22, 23, 24]. There are several studies on detecting misogyny
talks in social networks [25, 26]. However, there has not
been any study to identify incels based on their comments on
social media.
To identify incel members of social media, the only study
in this area has been carried out by Hajarian et al. [3], which
implemented Gegli social network users’ like and fuzzy like
[27] data to detect incels. This method has an accuracy of
23.21% using like data and 68.75% using fuzzy like data.
However, the users’ comments have the potential to increase
the performance of detecting incels in social media compared
with the previously used detection method. Therefore, in this
study, for the first time, we have proposed an innovative
method to identify incels inside social media based on
sentiment analysis on users’ comments. To reach this goal,
we have collected a dataset from two social networks and
make them publicly available for future studies by the
researchers.
Moreover, we have described the experimental results of
sentiment and offensive language analysis using our
proposed method. The effectiveness of the proposed method
in identifying incels has also been evaluated. The outcome of
this research can be used by researchers in different fields of
science for further studies. Social media and other online
communities companies can also benefit from the proposed
method to detect incels in their platform using their users’
generated comments or by using the open dataset we have
contributed in this study which is collected to help the
researchers make a better society and prevent further
problems that incels can cause to the citizens.
II. RELATED WORKS
This articles’ related works have been divided into two
categories: incels in social media and misogyny comments.
Although it is not possible to say if a person is an incel or not
just based on detecting misogyny comments, one of the
essential blocks of our proposed algorithm to identifying
incels is detecting such comments. As a result, in this
section, we will first review the related works about
misogyny comments, and then we will review the related
works about incels in social media.
A. Misogyny comments
To identifying the misogyny talks, Jaki et al. [28]
conducted a study on the Incels.me forum (which has been
suspended and is not accessible anymore).
NLP and machine learning methods to study the users’
comments and identify the misogyny, racism, and
homophobia messages inside user messages. Their proposed
deep learning method accuracy was 95%
Similarly, Saha et al. [29] developed a system using a
machine learning model to identify misogyny tweets in
Twitter with 0.704 accuracies. Using deep learning in [30],
the author proposed an approach by implementing a
Recurrent Neural Network (RNN) and ensemble classifier to
identify hateful content on 16k tweets to find sexism and
racism content.
In another study, Lynn et al. [31] conduct an experiment
to identify misogyny speech inside the urban dictionary.
They have compared the performance of two deep learning
methods with several machine learning methods, and they
found that deep learning methods, especially Bi-LSTM and
Bi-GRU, are more successful in deleting misogyny in the
urban dictionary. Although social network users’ comments
were not used in this study, similar to previous methods, it
shows the effectiveness of NLP and machine learning
methods in detecting hateful comments.
In [32] using social network analysis methods, Mathew et
al. reviewed the diffusion differences among hateful and
non-hateful speeches, and they discovered that hateful
speeches spread faster because hateful users in their use case
(gab.com) were more densely connected. This shows the role
of social network analysis in identifying incels.
Recently García-Díaz et al. [33] used sentiment analysis
in social networks for detecting misogyny comments. This
method was especially beneficial for comments in Spanish.
Authors find that by using this method, the accuracy of
misogyny talk detection can be increased.
B. Incels in social media
Identifying incels in social networks has been studied in
[3]. The authors proposed an algorithm to identify incels
based on the users’ like and fuzzy like data. Authors showed
that identifying incels using fuzzy like data is more effective
than using classic like data, and it has an accuracy of 68.75%
while using classic like data, their incel detection algorithm
accuracy is 23.21%.
Papadamou et al. [34] reviewed the youtube videos to
identify the incel communities. They found that there is
much inappropriate content generated on youtube by incels.
They found that not only has the content generated about
incels has been increased in recent years, the platforms such
as youtube also direct users to such content by
recommending them. As a result, social media should use
algorithms to identify such content in their platform and to
prevent incel rebellion, not recommend such comments to
their users.
In [35], the author conducted that the incel community
has become a place for young adults to avenge the girls who
rejected them, and these activities need to make changes in
the law of communication. Although forensic activities could
help reduce such activities, researchers should still look for
solutions to detect such problems in online communities.
Jaki [36] investigate the reasons for the 2018 Toronto
attack using incels.me and reddit.com websites by
implementing qualitative and quantitative content analysis.
They mentioned that after suspension of the incels.me most
of the users have migrated to Reddit. They have analyzed the
linguistic trends by harvesting textual data to understand
which words are most used by incels. However, the authors
did not use these findings to develop a method for preventing
such terrorist acts.
In [37], Rouda and Siegel describe why young men are
attracted to incel communities. Different reasons such as
loneliness and being angry about women is among these
reasons. The authors, however, only investigates the incel-
related terrorist acts, not detection methods. The author also
stated that before Elliot Rodger’s manifesto, misogyny
activities were much less. However, these type of activities
has been an increase in recent years, in this type of activities
are not organized crimes, but these are lonely people who
only need a friend or psychological counseling.
Another research by Helena E. Bieselt [38] conducted a
study to identify the personality features of incels using a
survey. The results suggest that in comparison with other
people, incels are less agreeable, extraversive, and
conscientious, and in contrast, they are more neurotic.
As reviewed literature in this section shows, NLP and
machine learning methods are trendy and can be considered
the primary solution for identifying misogyny comments in
social media. On the other hand, except the study in [3] for
identifying incels, other studies in these fields focus on
detecting hateful and misogyny content, not detecting incel
users. Because based on the incel definition in [3], incels are
male social network members who think women are not
attracted to them. As a result, if one wants to identify incels
inside users’ comments, s/he should consider more features
than just misogyny talks. The existence of profanity in the
text [39] and the gender of the commenter is also decisive for
this kind of detection. Hence, there is a big difference
between the misogyny content detection method and
identifying incels. Furthermore, to help incels and prevent
social problems, detecting incels is more critical than
detecting misogyny or hateful messages. As the literature
review shows, to this date, NLP and machine learning
methods were not used to identify incels in social media,
although they were implemented for detecting hateful and
misogyny content. Therefore, in this paper, we have
proposed an innovative method to fill this research gap. The
following section will describe our proposed method to
identify incels in social networks using text mining and
sentiment analysis.
III. MATERIAL AND METHODS
This section will describe our proposed method for
identifying incels in social networks using text mining and
sentiment analysis. We will also describe how we have
evaluated this method and what social network datasets have
been used consequently.
A. Proposed method
Fig. 1 illustrates the proposed method flowchart. The first
step was to read the user comments from datasets. Next, to
perform sentiment analysis, it was essential to preprocess the
collected data and remove unnecessary data. Thus, all the
punctuation marks, symbols, links, numbers, and spaces
were removed in this step.
After preprocessing, sentiment analysis has been
performed on the collected data. Based on the user
comment’s polarity, a variable called “incel” will receive a
value between -1 to 1. The negative polarity is -1, positive
polarity is +1, and neutral polarity is 0. If the polarity is
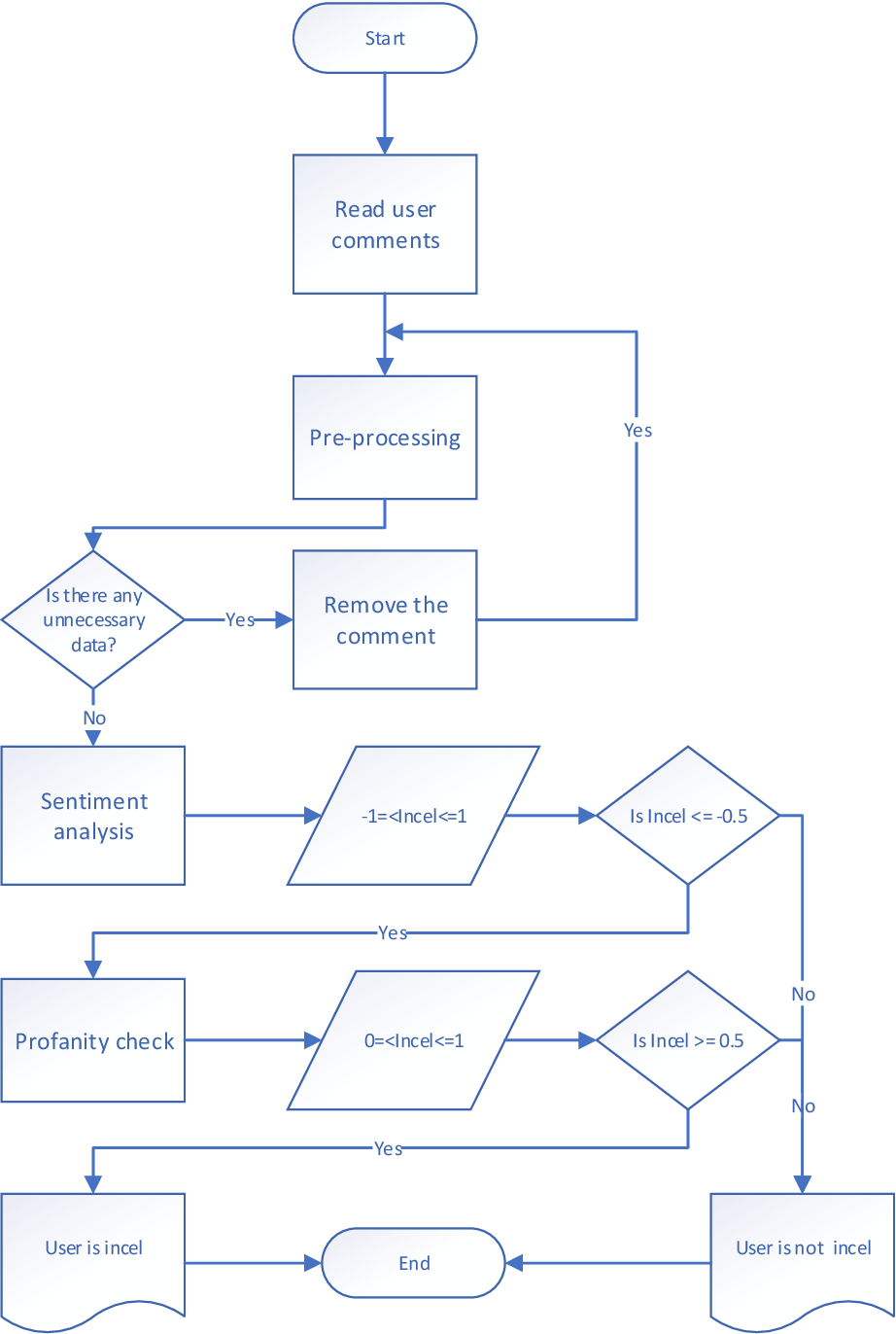
JFL at ''profanity check''
Fig. 1. Proposed method flowchart
bigger than -0.5, we decide this comment does not represent
an incel user. However, if it is equal to or less than -0.5, it
will enter the next stage.
In the next stage, we have checked whether there is any
offensive language inside the user comment. Based on the
result, the “incel” variable received values 0 for nonoffensive
language and 1 for offensive language.
After this, if the value is 1, we have identified the user as
an incel. Otherwise, this comment does not represent an incel
user.
To conduct the experiments, we have used windows 10
with Python version 3.6.9. For identifying offensive words,
the profanity-check library has been used and TextBlob for
sentiment analysis.
B. Dataset
In this study, user comments data has been collected from
two social media, namely Facebook and Twitter. We have
considered similar studies in collecting data for this study,
such as [31, 40]. In total, to perform this study, we have
collected 1048576 tweets from Twitter and 22058 Facebook
posts. After preprocessing the collected data and removing
unnecessary data and female users (because incels are male
users), we had 511367 tweets and 9146 Facebook comments
ready for the experiment. The datasets are available at
https://github.com/khanbabaloo?tab=repositories.
Moreover, to evaluate this method and understand how
much the proposed method is successful in identifying the
incels, 1081 incels comments were collected from Incels.co
for comparing the results with the actual incel speech. Then
the identified incel users based on the proposed method were
manually reviewed by the researchers to see if the comments
represent the incel user or not. The results of this evaluation
are described in the next section.
IV. R
ESULTS
In this section, the result of experiments using the
proposed method on each dataset along with its overall
accuracy will be described.
Based on the proposed method, we have performed
sentiment analysis and offensive language calculation on the
collected data from Twitter and Facebook after preprocessing
the social network user comments. Fig. 2 and Fig. 3 show
the results of implementing the proposed method separately
on each of the collected datasets.
As Fig. 2 shows, the proposed method has identified
9.1% of the users in the Facebook dataset as incels.
Also, Fig. 3 shows that the proposed method has
identified 8.5% of the Twitter dataset users as an incel.
To see how much we have correctly identified incels
considering the collected data from different social networks,
we have manually reviewed the analysis results with respect
to the collected comments from Incels.co website.
Fig. 4 shows the results of this review and the accuracy
of the proposed method. As it can be seen, the review result
showed that the proposed method was able to identify 78/8%
of the incel users correctly. In contrast, 21.2% of the users
were not identified correctly.
V. C
ONCLUSION
In this paper, we reviewed related works about detecting
incels and misogyny speech in social networks. Moreover,
we have proposed a method to identify incels in social media
for the first time by analyzing users’ comments.
Accordingly, sentiment analysis and NLP methods were used
for detecting offensive language in this study. Experimental
results show that the proposed method is able to identify
incels in social networks based on the user comments with
78.8% accuracy. Compared to previous work in [3], which
has used fuzzy like data, this study’s proposed method has a
better performance of 10.05% in identifying incels in social
media.
Nevertheless, this method used a completely different
approach to detect incels in social networks. Hence, we can
not say that this method is a substitute for the proposed
method in [3]. However, both of these methods can be
beneficial in specific conditions. Because one of them is
based on the social network users’ like and fuzzy like data,
and the other is based on the social network user comments.
Both of these methods can also work as complementary for
each other to generate more accurate results.
However, this field of study is emerging, and many other
solutions can be considered for further experiments. For
future works, we suggested using other machine learning
methods. In [31], the authors found that the Bidirectional
Long short-term memory (Bi-LSTM) method has the best
performance in identifying misogyny talks. As a result, using
the Bi-LSTM method can be a subject for future studies in
this area. Moreover, for future studies, the social analysis
methods can also be considered. As mentioned in [32],
hateful speech spread faster, and their publishers have a more
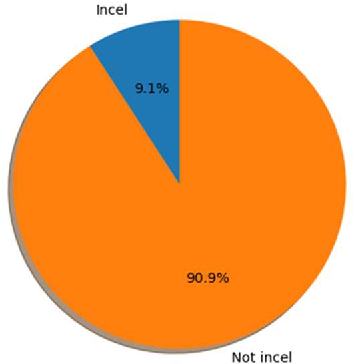
Fig. 2. Percentage of identified incels in Facebook datasets.
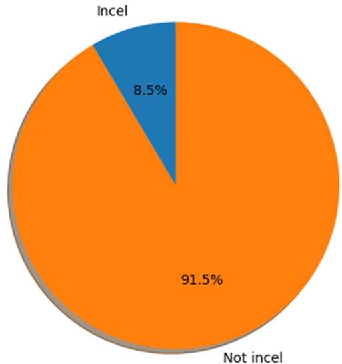
Fig. 3. Percentage of identified incels in Twitter datasets.
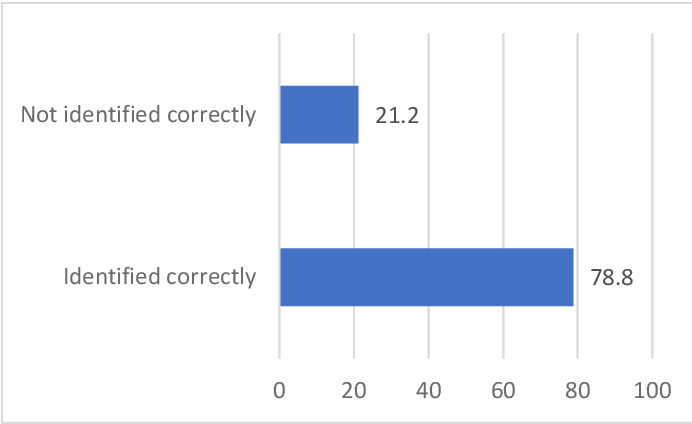
Fig. 4. Proposed method accuracy
dense network. Hence from a social network analysis
perspective, the position of users in the network should be a
helpful piece of information for better identification incel
users. Furthermore, after detecting incel members,
gamification, which implements game elements in no game
context [41, 42, 43, 44], can be beneficial to encourage incels
members to participate in more constructive talks. However,
it should be used with appropriate interaction design for
social networks [45, 46, 47]. Processing other media types,
like the posted image, video, or audio files [48, 49, 50] by
the users, can be subject to further studies in identifying
incels in social media.
good luck finding me on jewbook morons, the only ''social media'' i use is this site, over before it began for tHe InCeL ReBeLlIoN jfl
they should develope a system to help identifying inceltear's pedos instead tbhngl